Pharma’s big data opportunity
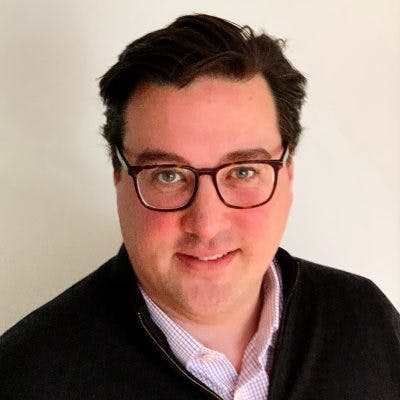
Pharma’s big data opportunity
First world health and care systems went into the pandemic with big structural issues. These issues have grown significantly because of Covid, but Big Pharma has an opportunity to move before being compelled.
Fatigued health workers now expect improved pay and conditions, indebted states need to radically reduce spending yet drug costs keep increasing and the backlog in non-pandemic activity must be addressed even though countless patients will now die before being treated. That is merely the tip of the iceberg.
This is uncomfortable for big pharma. The historic business model was quite simple and highly profitable. High-value drugs sold for good prices with lengthy patent protection.
Change is afoot
The new model will need to combine increasing delivery complexity with local data on patients and populations to demonstrate public value – outcome-based payments. This transfers a host of new risks to the pharmaceutical company and demands new capabilities to price and manage them, while also increasing the number and range of commercial agreements.
We have already seen the start of outcome-based pricing, such as Novartis’ Zolgensma at ~$2m per patient to treat spinal muscular atrophy. And these moves are welcome in the context of targeting life-changing and life-ending diseases. But so far this remains very ‘narrow’ in terms of patient data and use cases and does nothing to deal with the politically-charged issues of population health outcomes, especially the widely reported discrepancies across socio-economic divides.
Applying data science
Orienting into this new landscape requires data: the ability to generate robust medical and commercial insight by combining many different individual and population-level sources.
Everyone wins through better analytic insight, forecasting and the experience of working together, building a bridge towards the outcome-based commercial mechanisms of the near-term future. Yet pharma companies have shied away from holding patient data, outside of narrow technical research fields.
A first step would be the use of existing machine learning and data science to improve expensive drug targeting. Federated learning allows AI-training and pattern recognition across different data sets without sharing the data, and ‘deep fake’ methods offer entirely fabricated information that closely mirror characteristics of the original.
These approaches allow different organisations (and divisions within the same global company) to come together and collaborate, whilst controlling overall data risk exposure. Such steps can allow piecemeal collaboration over time, benefitting from new information as it becomes available. This includes enabling and seeking patient sharing of information from ‘smart’ wearables.
These approaches offer big pharma companies a stepping-stone: building alliances, generating trust, and even building competitive positions and patient-accessible data-sharing platforms. But deeper disruption is coming from data-rich tech giants. They are already working towards their first £-trillion in annual health revenues.
About The Author
Alasdair Ramage is a director at Arca Blanca. He specialises in modernising the way organisations are run: using data to drive performance, shifting operating models, transactions and digitisation.
About Arca Blanca
Arca Blanca is an integrated consulting and data company, resolving complex growth and profitability challenges. We help businesses of all types thrive in the face of unprecedented change and upheaval driven by new technologies.